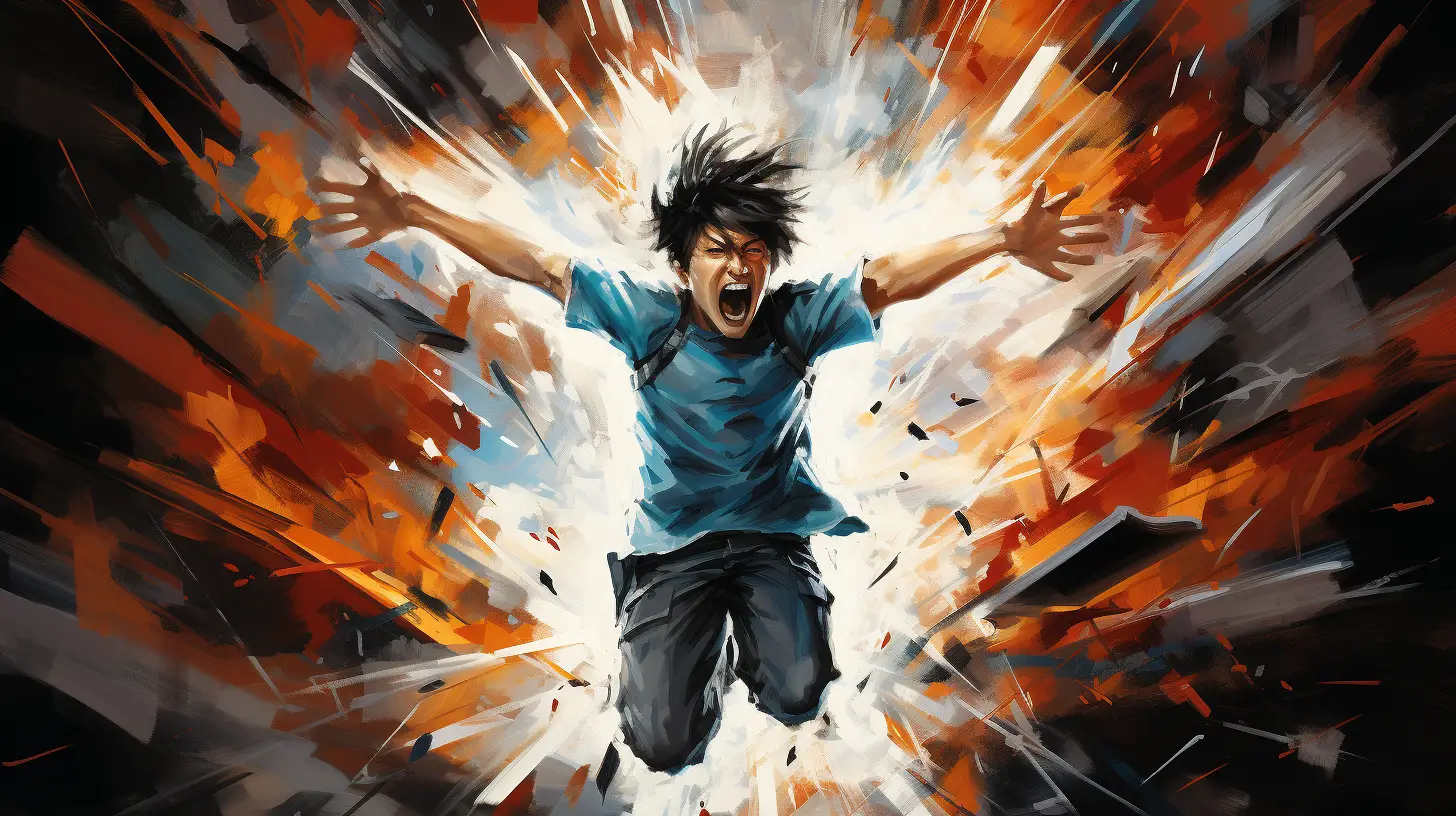
Understanding Sentiment Analysis: The Key to Emotional Insights in Text
Sentiment analysis is similar to peering into the human psyche through the lens of text. It's not just about numbers and algorithms, it's about capturing the whispers of sentiment floating through the vast ocean of data. As we jump into this world, you'll see how necessary understanding emotional nuances is not just for smart corporations, but for anyone venturing to make sense of opinion and emotion in text.
The influence of sentiment analysis transcends industries. From marketing teams fine tuning their ad campaigns based on real consumer feelings to political strategists gauging public sentiment before elections, the versatility is astounding. Even customer service departments are leveraging this technology to strengthen their response times based on feedback. A wave of evolution is sweeping through businesses, making sentiment analysis an indispensable tool for anyone who dares to connect with their audience.
The Evolution of Sentiment Analysis
Let's rewind to a time when sentiment analysis was fresh on the scene. Initially, it was mostly a scramble, relying on rules deeply integrated into text. Early applications often fell into the black and white recognition of positives and negatives, much like flipping a coin. But as technology advanced, the context changed. Enter machine learning. Complex algorithms began to ease their way into sentiment analysis, providing a deeper understanding that mere rules could never deliver.
Important milestones such as sentiment aware tools emerged, revolutionizing how we extract meaning from text. Take the introduction of SentiWordNet, for instance, which brilliantly mapped out sentiment in a user friendly format. It was a major breakthrough. Fast forward a few years, and we're knee deep in neural networks that can examine context, tone, and even nuances like sarcasm a feat once deemed impossible.
How Sentiment Analysis Works
Before we conquer the world with sentiment analysis, the text needs a makeover. That's where preprocessing comes into play. Think of tokenization like slicing a loaf of bread into manageable pieces, while stemming and lemmatization polish it up for consumption.
Now, let's break down the methods used to dissect emotions. Vocabulary based approaches are the sturdy old friends of sentiment analysis. Using predefined dictionaries, they lay down a foundation that's simple but effective. Enter machine learning models like Naive Bayes and Support Vector Machines, which step in to tackle emotional complexities in larger datasets. And as the grand finale, deep learning techniques, utilizing neural networks, come to the rescue. They can grasp subtleties humans might overlook like when someone says, “Wow, that's just great!” dripping sarcasm.
Applications of Sentiment Analysis
How does this power play out in the real world? Well, in marketing and brand management, companies are discovering deep currents of consumer sentiment that shape their strategies. It's like having a conversation with your audience that's both insightful and illuminating. Brands are not just reacting, they're anticipating needs based on the emotional environment painted through data.
Social media monitoring takes this a step further, providing a live pulse on consumer attitudes. Imagine managing a crisis before it spirals out of control by understanding real time sentiment changes. Political analysis benefits too, campaigns can pivot based on voter sentiment instantly, adapting their message to relate more deeply.
Look closely at customer feedback, sentiment analysis is a trusty companion here. By deciphering emotions hidden in feedback, companies refine their products and services, turning complaints into enhancements.
Challenges in Sentiment Analysis
But hold on! This magic trick isn't without its hurdles. The beauty of language is its ambiguity, making accurate sentiment identification a challenge. Just think of idioms, they can swing meanings left and right. Sarcasm is the rogue element that keeps analysts up at night how do you classify a “great job” when it's laced with irony?
Besides, industries often speak their own language. What delights a tech guru might baffle someone in healthcare. As such, general sentiment algorithms don't always perform well across domains, making bespoke solutions necessary.
Future Trends in Sentiment Analysis
What's next on this wild ride? Advancements in AI and machine learning are poised to push the envelope even further. Imagine systems evolving in how they learn behavior from historical data spooky but impressive! Sentiment analysis will increasingly hold hands with other analytical tools, creating an ecosystem richer in insights than ever before.
Although, we've got to keep our ethics compass true. As we harness this power, privacy and ethical ramifications must remain key considerations. Dissecting emotions from data is one thing, doing it responsibly is another.
Tools and Resources
Ready to jump deeper? Several popular sentiment analysis tools can ease your entry. NLTK and TextBlob are like trusty sidekicks for many starting out, while tools like MonkeyLearn offer solid solutions with added flair. Each option has its pros and cons, and finding the right fit is essential for success.
The Future of Sentiment Analysis
What lies ahead for sentiment analysis? Will technology grow to predict societal trends before they happen? This imaginative notion could reshape our understanding of human interactions. If the algorithms evolve, can we foresee moments when sentiments collide, sparking movements before they catch fire?
So here's the deal: your experiences matter in this conversation. Consider how sentiments influence your everyday interactions and decisions. Navigating the complexities is a shared journey, and the future can only get more intriguing.